Simplifying HRTF measurements using machine learning
New SONICOM research has shown that machine learning approaches can be effective at spatially upsampling head-related transfer functions (HRTFs), outperforming other methods when very few measurements are taken.
Published in IEEE Explore, the research could make it easier to create high resolution HRTFs by reducing the number of necessary measurements that have to be taken and using a neural network pre-trained on existing HRTF datasets to increase the spatial resolution.
“Traditional methods [of upsampling], such as barycentric and/or spherical harmonics-based interpolation, can only rely on the data they are given to upsample, therefore in very sparse conditions performances are very much related to the number of measured positions,” said Dr Lorenzo Picinali, SONICOM’s lead PI based at Imperial College London.
“A pre-trained neural network such as the one we designed, on the other hand, can use also the data it has been trained with, resulting in significantly higher performances in very sparse conditions.”
Democratising HRTF measurement
An HRTF is a mathematical way of describing the unique way someone’s ears receive sound depending on the position of the sound source. Because everyone’s heads, ears and shoulders are different sizes and shapes, the way that sound waves are modified when they reach our eardrums is different from person to person.
Measuring your individual HRTF means it can be used to make sound coming through headphones feel more realistic with accurate distance and direction. For this reason, HRTFs are very important for creating realistic virtual reality (VR) and augmented reality (AR) environments.
However, the best way to measure high-quality HRTFs requires expensive equipment, bespoke lab environments, and the recording of thousands of measurements.
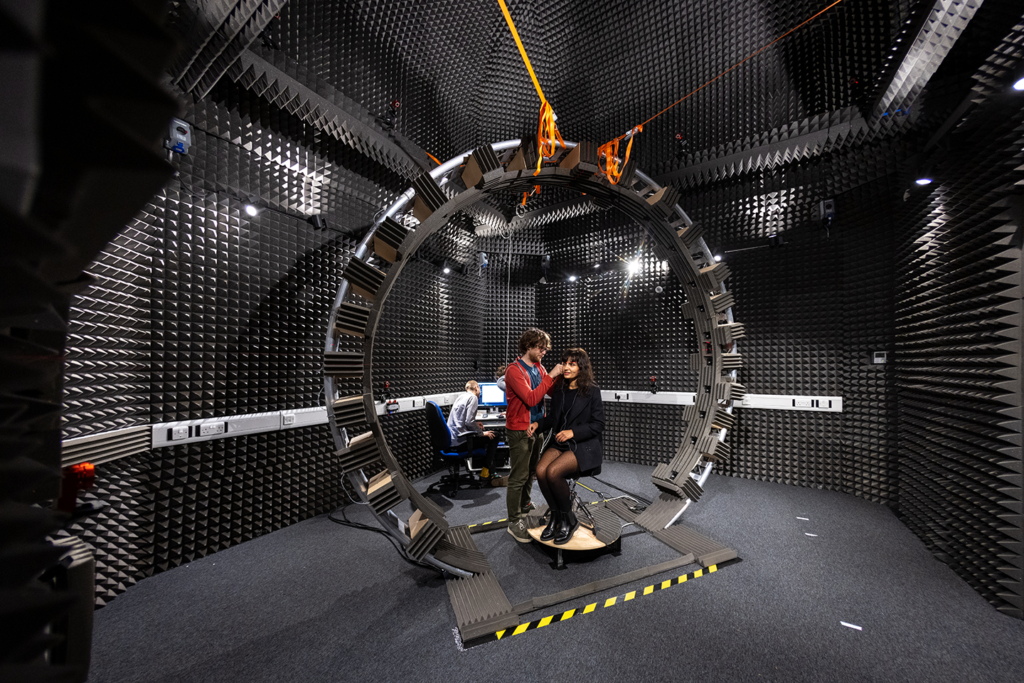
The Turret Lab at Imperial College London showcasing the equipment required to acoustically measure an HRTF.
To overcome these limitations, work has been done to see if we can ‘upsample’ low-resolution HRTFs that are made with fewer measurements into high-resolution versions – like recreating a detailed painting from just a sketch.
This new research harnesses a type of artificial intelligence (AI) called Generative Adversarial Networks (GANs), which has been successfully used in the past to upsample low-resolution images. After having trained it with data from the SONICOM HRTF dataset, when provided a low-resolution HRTF (e.g. 5-10 positions only, compared with the hundreds of the full resolution version), the GAN can create a high-resolution version.
GAN-upsampling was shown to perform better than other upsampling techniques when dealing with very limited data.
If upsampling techniques can be improved, it could make it much easier to produce high-quality HRTFs – perhaps even from simple recordings done in the comfort of your own home.